What's the value of your data products?
How fluent are you in speaking the language of your business? 🤑
As (Data) Product Managers, we’re very accustomed to using prioritisation frameworks to decide what should be worked on now, next, or later on (if ever). From the simple but great Impact vs Effort 2x2, to more elaborate models like ICE, RICE, and WSJF, there’s a lot of techniques to help us take a systematic approach to prioritisation, rather than just do it based on the salary or title of the person asking for something.
We also don’t overcomplicate the art of prioritisation into a full-blown science. We want prioritisation to be quick and easy, especially when doing a first-pass scan that’s about zeroing in on the top x% of the work, and parking the rest either in a backlog or trashcan. So, if we’re taking “effort” as one input, that won’t be a full-blown work breakdown structure for each new feature, estimated in # of FTE hours and other resource requirements. It’s more likely to be a t-shirt size (small, medium, large, extra large) or maybe a score ranging from 1 to 5. Same for impact, confidence, reach, or any other proxy for those things you might be using.
This makes sense if your product team is empowered - meaning you don’t need to be micromanaged/get approvals from above every time you want to work on a new feature. It also helps if you have a reasonable ability to get other resources you might need - a data engineer’s time, some third-party data, and keep stakeholders who want to work with you to build things to help them.
In many ways, working in such a team and org is a very big green flag. It means your leadership gets that the 1900s hierarchical model of management is not conducive to modern knowledge work, where seniority doesn’t equal expertise, and self-organising product teams can deliver enormous value without (much) top-down direction.
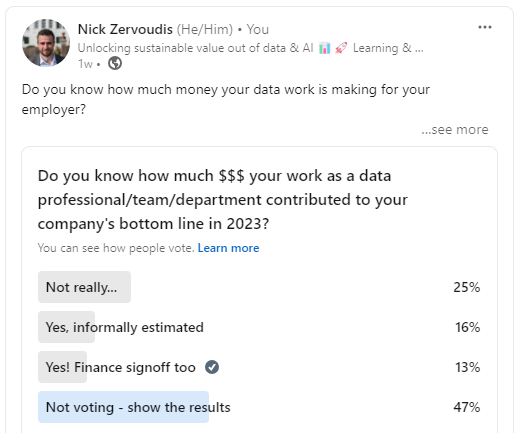
In our quest for simplified prioritisation, we sometimes forget the language of business
Not having to worry too much about “buy in” and “business cases” can also be a trap for data teams. Consider that many data teams have operated for years with a relative carte blanche - a blank cheque to work on what they think will help the business. After all, data scientist is the sexiest job of the 21st century, and data is the new oil, right? And yet, this has helped engender a “build it and they will come” mindset that’s left with data products not being adopted or delivering value, or with data teams simply beholden to HiPPO request after HiPPO request, or with endless projects to build “data foundations” and platforms and then move to the next shiny platform tech before the old one even started being used in anger. It’s no surprise that we see study after study about how data teams fail to provide ROI-positive value to their organisations (e.g. last year Gartner reported that less than half of data and analytics teams provide value - as reported by their own leaders)
“Just because I don’t do long business cases for every new feature or product doesn’t mean I just think I should build it and they will come”, you might (very rightly) be thinking. I agree. Sometimes a simple ICE T-shirt exercise is enough to identify clear winning bets, and the time it would take to validate those assumptions is better spent actually building the damn thing. That’s because sometimes it’s obvious enough how a new project/product/feature/whatever is going to result in a big uplift in sales, or cut costs, or whatever else matters to your business most, and all without costing a fortune in time and money.
For example, maybe a single sale of a new external-facing data product will bring in $1M, and you know this because you’ve got a client ready to sign. You also know it’ll cost you at most $100k (unrealistically conservative estimate) to build and maintain, so you’re happy to put this as the #1 priority for this quarter - nothing else has a 10-20x ROI projection at the moment. So you’re not concerned about the specifics of cost to build, or whether you’ll be able to scale the product to more customers.
We can project this example into a broader 2x2 matrix looking at expected impact vs cost:
The above assumes a reasonably high confidence about costs and impact/value. If you're not sure yet, you have two options: (1) Accept the risk, or (2) spend more time validating your assumptions.
Here's what this year's potential work might look like on this 2x2:
Do you know your data team's ROI?
I'm willing to bet you've got gaps. A lot of data initiatives, especially the ones that are internal to an organisation, often have a very ambiguous returns - or at least they're not reported by and known by the data team itself. Finance or Commercial might have some numbers somewhere that overlap with your work, but it won't quite be 'ROI per data product'.
Similarly, you might be missing the cost side of things - even if you have an idea of value generated, at least from some initiatives.
Here’s a few examples of where your assumptions might be lacking on the value (or impact, or benefit) side of things:
Your benefit is very simple to describe: This is a request coming from the CEO/CIO/SVP of Marketing/other HiPPO, so the impact of the request is you’ll keep this person happy (or at least not mad at you) 🙃
You know how many FTE hours you’re going to save/unlock, but don’t know how much those are worth to the business. You can’t go around asking people for their payslips, can you?
You have an idea for how much your ML model could improve a metric by, but there’s so many dependencies… You don’t want to commit to a target before version 1 has been built.
Even if you know with certainty that you’ll e.g. predict churn probability with an accuracy of x% or higher, you don’t own the actual outcome, which is the sales team using these scores to prioritise which customers they call and offer “please stay with us” discounts.
Maybe you’re confident that you and your stakeholder/sponsor in XYZ department will co-own the outcome, because you’re building an end-to-end product, not just a black box model, but neither of you is sure how to quantify the impact in monetary terms. But the operational metric is widely-known around the business, so you’re happy to articulate your product’s benefit in terms of that KPI.
And on the cost side:
You treat the labour cost of your team as free, because it’s a given. We’re gonna pay them regardless of what they do, right?
You don’t know exactly how much compute, storage, and other cloud costs your product is costing at the development or production phases. All you know is maybe how much your whole department's cloud spend is (and you don’t like thinking about such big red numbers)
You ignore any cost that your stakeholders’ departments will incur, like the FTE hours they need to spend to help you build something for them (except maybe when it comes to calculating how many FTE hours you’re saving them!)
If you're missing the above, you can't know where on the 2x2 a new request or existing product sits! And even if you have some idea about it, it'll be harder to communicate that 2x2 position to others, like the HiPPO requesting dashboards that are a waste of everyone's time.
OK, I get it. Knowing the dollar value will help me prioritise what work to do first, next, or never.
It does! But that's not all.
Internal buy-in: Putting hard numbers against requests for resources makes the conversation much more likely to go in your favour (at least if your request makes financial sense). Maybe you need another data engineer, or a software license that'll drive your team's productivity up, or more love from marketing, or budget to buy third-party data... It all costs money at the end of the day, and that's all it is when you look at it from Finance's point of view.
Client buy-in: If you're selling to external clients, you're gonna benefit greatly from this sort of quantified benefits analysis. If you know the client does £50M in annual revenue, and your product can demonstrably help raise that by 1% 6 months after they buy, that's £250k in year 1, and £2.25M by year 5. Not too bad for a £100k/year subscription fee, right?
Your own progression and recognition at your company: Your end of year review is going to look a lot more positive if you can demonstrate in no uncertain terms the business value you and your team have added.
A better CV/resume for when it's time to move on: Being able to highlight your impact is (a) a very important thing one's CV should always be doing, but (b) especially important if you're a PM, where the ability to do so is a key skill for the job itself. A bit like a web developer with a videogame-like CV that showcases their skills (just not as exciting).
OK, OK, fine. I know quantifying impact is valuable. But for my product, it's uniquely hard, you see...
You're probably thinking this, aren't you? I know I have too. Lots of times. It's hard, but not as hard as you think. And, like many hard things, it's very much worth it. If it was easy, it probably would've been done already...
I want to write another piece going in depth on how to do all of this, but until then, here's some tips that'll get you 80% of the way there:
Keep it simple. Don't expect a scientific, high-precision measurement. Especially for version 1. Like with other parts of the job, we start simple, and add complexity over time - if need be. If you only had 15 minutes and a piece of paper to do a back of the envelope calculation of the benefit, what would that look like? Start there. Document your assumptions.
Involve others. Just like you're not expected to write the code for your product, or run sales, or fulfil deliveries, this too is a team effort. Maybe you need to get input from your technical users to understand how much more accurate their models are thanks to your data. Or maybe it's about speaking to operational users to understand roughly how much quicker they're taking a decision now that they have a dashboard instead of a pile of Excel sheets flying around. Or you need to speak to Finance to get a rough idea of how much each FTE hour or litre of fuel saved is worth to the business.
Get signoff. It's one thing for the data team to report that they helped generate $50M in sales, save £200k in fuel costs, or prevent a €1M fine, and another for those numbers to be fully credible (and credited!) by the business. Involve Finance or whoever it is that can back these estimates. They'll probably also help you with using the right assumptions and simplifying them enough, but not too much.
Do all of this before the work, not after. It's going to be much easier to get signoff without being accused of moving goalposts or trying to get credit retrospectively for an outcome you didn't influence. Most importantly, it's going to be so much easier to estimate the value of a product if there is a clear proposition for how it's going to add value. If you don't do this before development starts, there is a much higher chance you'll end up working on the wrong thing, and THAT'S why it'll then be much harder to quantify the value. Built a dashboard an exec asked for once and then never looked at? Yeah, I have bad news for you...
Conclusion
The best time to start estimating the potential cost and value of a data product, project, or team is before the work starts. The next-best time to do this is right now. Start simple, start crude, start with the larger projects and forget about the long tail of dashboards and small projects for now. Maybe that's enough to get you the resources you need to stop being so under-staffed, under-tooled, or under-appreciated. Or maybe it's the first step.
Further reading
The approach I've described in this article is aimed at DPMs within existing orgs and operating models. If you're a data leader in a position to establish a broader governance process,
‘s latest article offers a fantastic blueprint. It's one of those "I can't believe he's giving this out for free" articles you should bookmark and reference.Again by Benny is a great heuristic for determining what % of the value should be credited back to the data team. He's covered it in a few places, but my bookmark is to minute 11 on this podcast.
On 'starting simple', a great technique is Fermi estimation. The idea is you're looking to get the order of magnitude right using back-of-the-envelope calculations, not arrive at a super precise figure. Ultimately, knowing how many zeroes you can expect on the cost or benefit side is usually going to be enough to make a decision.
Other news and events I wanted to share
We had our fifth and largest-ever London data product management meetup last month 🥳 If you want to get notified of future meetups, sign up here.
I've opened up free coaching calls! If you or someone you know would benefit from a chat about breaking into data product management, or for those already in the trenches looking to get an outside perspective, you can book a call here.
Orbis have launched a new podcast, Product Leaders, whose inaugural season had not one, but two data product episodes! Check out my London DPM meetup co-host Caroline's episode here, and mine here.
On a final (slightly too self-centred note for my liking) note, I met with Harbr's Anthony Cosgrove and IAG Loyalty's Chanade Hemming to talk about data product management. You can find the webcast on YouTube.
There’s a LinkedIn live happening tomorrow (13/03) I’m very excited about: Jon Cooke, Stuart Winter-Tear, and William Alvarez-Garzon are getting together in Mindfuel’s latest DPM live webinar. Here’s the link to watch it.
Jon is also starting a podcast, Data Product Workshop. I’m looking forward to episode #1 on business storytelling that’s coming out next week (link).
I hear there’s another data product management podcast coming out soon… Watch this space 👀
Love this article, great read Nick! I always do the value vs. effort scale and I like how you framed it as impact (it just sounds so much more impactful)
I‘m so happy you started this topic and most importantly your newsletter, Nick!
Great insights. And this is the essence: „And, like many hard things, it's very much worth it. If it was easy, it probably would've been done already...“
Measuring impact all the times is the only thing what justifies your data initiative!!