The double-edged sword of having PMs in data science teams
Is specialisation of data roles always a good thing?
Something that's been bothering me for as long as I've been a Data PM is the feeling that I'm stunting the growth of my data scientist teammates. This also applies to other data roles (data engineer, data analyst), but as I work primarily with data scientists, I'm focusing mostly on that role in today's newsletter.
An (extremely brief) history lesson
In the early days of 'data science', you had unicorns that were seemingly able to do it all: Find the right opportunities, get the data, wrangle the data, build models, recommend decisions, productionise solutions. Or so the story went anyway.
As more companies started buying into the hype of the "sexiest job of the 21st century", we saw much-needed specialisation emerge: Data engineers started preceding data scientists, helping reduce the amount of time spent wrangling data (and doing it in a more scalable and sustainable way). Then came machine learning researchers, machine learning engineers, model analysts, data science product managers, analytics engineers, and so on.
Why do we specialise data roles?
The logic is the same, or at least similar, as in any scaling organisation: What starts as a 1-person role (e.g. 'marketing manager') becomes a small team (e.g. a content marketer, SEO expert, and their manager), and gradually turns into a department comprised of many teams and specialisations (e.g. Brand / Growth / Acquisition). It's partly because the workload increases, but also because specialists have a deeper focus and expertise. If you're in the early days of forming a data team, you may not need someone who is an expert at fine-tuning ML models, or who can optimise data platform costs, or who has deep domain expertise in marketing mix modelling. You're just trying to get quick wins and often incur a lot of tech-debt to do so (which is good, given the alternative would be to over-engineer stuff you don't even know is needed).
I often see organisations see the Data PM as someone that can act as a shield for the rest of the data team: The person that can go to meetings, talk to people, think about what the team should work on while everyone else is focused on delivery. When your data scientists are complaining that they have so many meetings there's no time to do actual work, that seems like a good thing.
But it's a double-edged sword, because it's good for your data scientists (or analysts, or engineers) to be spending some time with end users, both to understand the problem space better, relate to the users and their pain points, but also to then provide suggestions as to what the solution should look like.
This is an issue on a few levels:
We might miss out on the right solution: When your technical team is there as you learn about the as-is process, the current way of doing things, the pain points, and who the users are, they might be able to put 1+1 together where the Data PM would've missed something. Maybe the DPM was going to propose something more complex than what's needed, maybe they'd have failed to identify a potential pain point as resolvable, maybe they'd not think a certain bit of information was worth passing on back to the team when they debrief.
It becomes harder for the data scientist/analyst/engineer to understand the impact of their work: It's one thing to be given context in a sprint planning session or via a Jira ticket, and another to actually shadow the person you're gonna help make better decisions, or whose tedious workflow you'll help simplify. If you're just executing tasks because they're on tickets with your name on, work gets less interesting and satisfying.
It stunts the data team member's professional development: Communicating with business stakeholders, identifying ways data can drive efficiency/growth/decisions, and understanding your org are all things that data scientists should be doing, and getting better at over time. That's how they'll progress to Senior then Manager, or move laterally to Data Product, or eventually become Head of Data.
How can I avoid stunting my teammates' development?
First off, talk to your technical teammates. Some will express the desire to spend more time with business stakeholders, or to take turns presenting, or maybe they'll want to give project management a go. Others will want to avoid as many meetings as possible, because they want to remain technically-focused ICs. Not everyone wants to make manager, or become T-shaped, and usually orgs need both profiles to succeed. Also, make sure to have this conversation more than once - people change their minds and priorities! I try to do it 1-2x a year usually. It also helps do things like allocate tasks or move folks across teams when you know what they actually want to do vs. what they do somewhat begrudgingly.
For the folks who want to get more business exposure: By all means, spare them from calls where you don't think they'll learn or contribute. For example that'll often be calls about project status or resourcing (though not always). Also feel free to agree on trials/experiments together. The grass is always greener on the other side, and some data scientists who at first say they want to be in more meetings or present or interview may change their mind after doing it - at which point they'll be grateful you're covering for them, rather than build up resentful thoughts.
Ensure your technical lead is your peer, not de facto subordinate. This might be the DS Manager, or they might just be the most senior data scientist or engineer on the project. This is a general good practice for many reasons, but in this context, they're the person that should be leading on Opportunity Discovery alongside you. As a rule of thumb, I aim to have my technical lead attend >80% of sessions that are about understanding either the problem space (and > 90% of sessions that are about fleshing out the solution).
A common working pattern from the software world is to have a Product Trio (Product Manager + Engineering lead + Designer) handle Discovery, and for the Eng/tech lead to then be responsible for briefing the technical team, breaking down the work, and managing delivery. A lot of data teams take a much more hierarchical approach, where the data lead / data product manager / data product owner isn't just covering the product side of things, but is also expected to act as the technical lead - even though they're often not technical enough to do so. Even if your Data PM is technically savvy, having two brains work on the same problem is usually better - as long as you're still clear about who owns which part of the work, rather than get to the classic "if there's more than one owner, then no one's owning anything" situation.
The last idea to throw out there is to give data scientists or data engineers some limited PM duties. At the end of the day, not every component or piece of work will end up being big enough to warrant having a DPM own it. Having a member of the technical team that built it also act as its owner will give you the dual benefit of (a) ensuring someone is doing things like maintaining the component, getting feedback, acting as the first point of contact, and over time improving it, while (b) giving that person a trial run for what it's like having to do that sort of thing. I will admit, this is something that we've often said we wanted to do, but it's not always stuck - and that's happened at every single company I've worked at since becoming a product manager 😅. You've gotta walk the walk, not just talk the talk! If someone's the lead/owner for a component, they need to be given the time to carry out that role - not expected to magically find 0.5-1 FTE days a week out of their free time.
Lastly, take note at how I'm talking about 'teammates' here, not reports. Even if organisationally you're a PM with the DS/DE folks on your team/product reporting to you, it's important to treat them as peers when it comes to their expertise.
How do we move ahead?
I no longer worry that having Data PMs in well-organised data product teams leads to the stunting of technically-focused team members. More junior (and/or antisocial) team members start off by focusing on delivery, and over time get the option to spend more time on business-facing tasks like discovery, planning, getting feedback. Technical leads get to be the DPM's peer, and bear equal or at least equivalent responsibility in validating opportunities and making sure their team is working on the right things, not just that they're working in the right ways.
But my worry remains for the teams that aren't really working as cross-functional product teams, but that have rather renamed their data project managers into "DPM" or "DPO" (usually it's the latter, and combined with a horrible, anti-Agile implementation of 'aGiLe' like SAFe). In such teams, you have a disconnect between the DPO/DPM and the technical team, and in turn between the business problem and the folks working on the solution for it. If you're a Data PM or PO in such a team, try involving your tech lead / some of your technical team in key meetings with your stakeholders.
If you're a data scientist feeling wrongly left out of meetings with stakeholders, try and suggest changing that, even if it's framed as a limited time trial. Point to examples where your non-involvement led to errors, delays, or other issues - but try not to frame it confrontationally. “What if we tried…” tends to work much better than “see, this is why I was pushing to…”, as it avoids putting the other person on the defensive.
Good luck!
Unrelated news from this month: DPM events
This month was busy on the IRL meetup side, with Data PM meetups taking place in Barcelona, London, and Montréal all days apart from each other. Plus, right after the London meetup came two days of BigDataLDN, one of the largest data conferences of the year. Data products were everywhere at BDL, though a lot of the time people were actually talking about the data-as-a-product mesh term (about which I’ll share a rant another day). Suffice to say, I'll be spending the next few days drinking smoothies, eating salads, and avoiding (too much) social contact to recharge my social and physical batteries 😆
Coming up:
September 24: Montréal Data PM meetup #3 (in person)
October 1: Mindfuel Value Measurement DPM panel with
, Andreas Mazat, William Alvarez, and myself (online)
I hear there might be a few more DPM meetups being organised in a couple of European cities… Watch this space 👀 Next week’s newsletter will touch on this some more, but if you’re thinking of organising a DPM meetup in your city, hit me up! I’d love to help out.
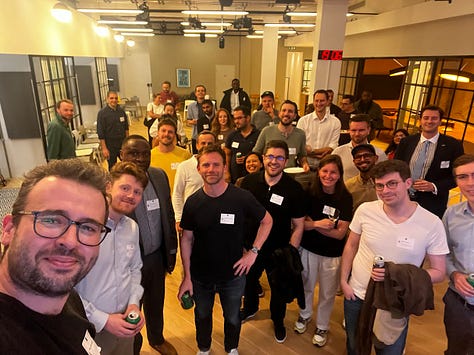
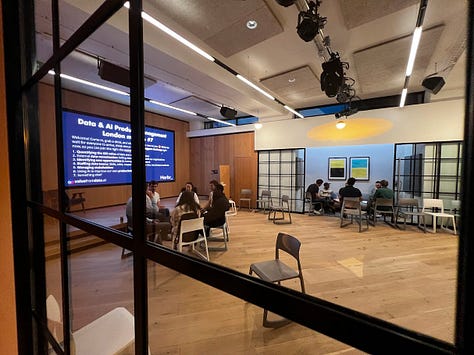
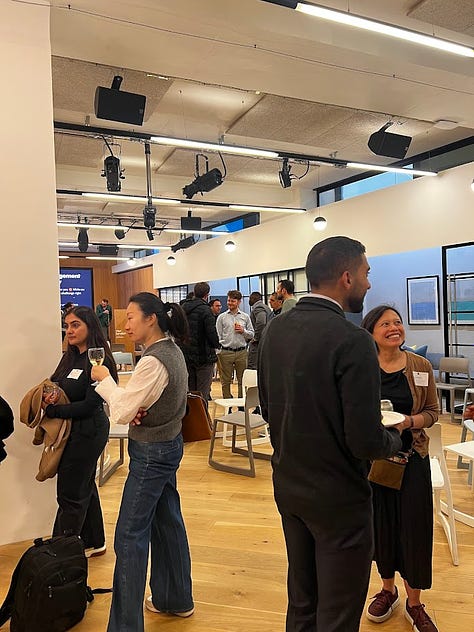
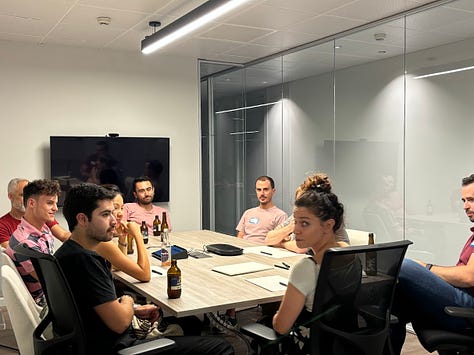
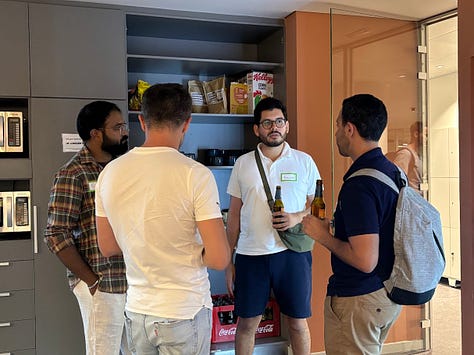
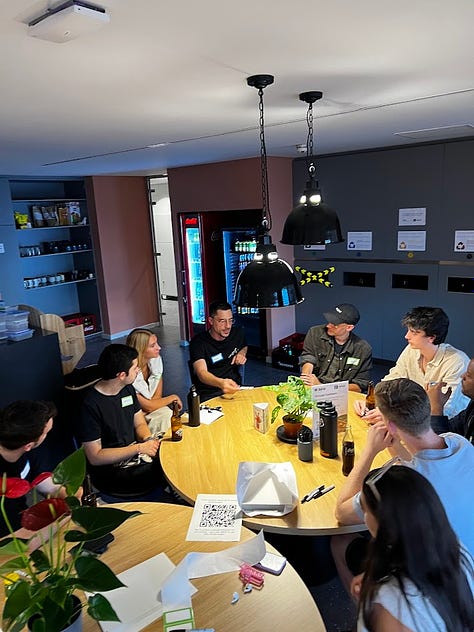
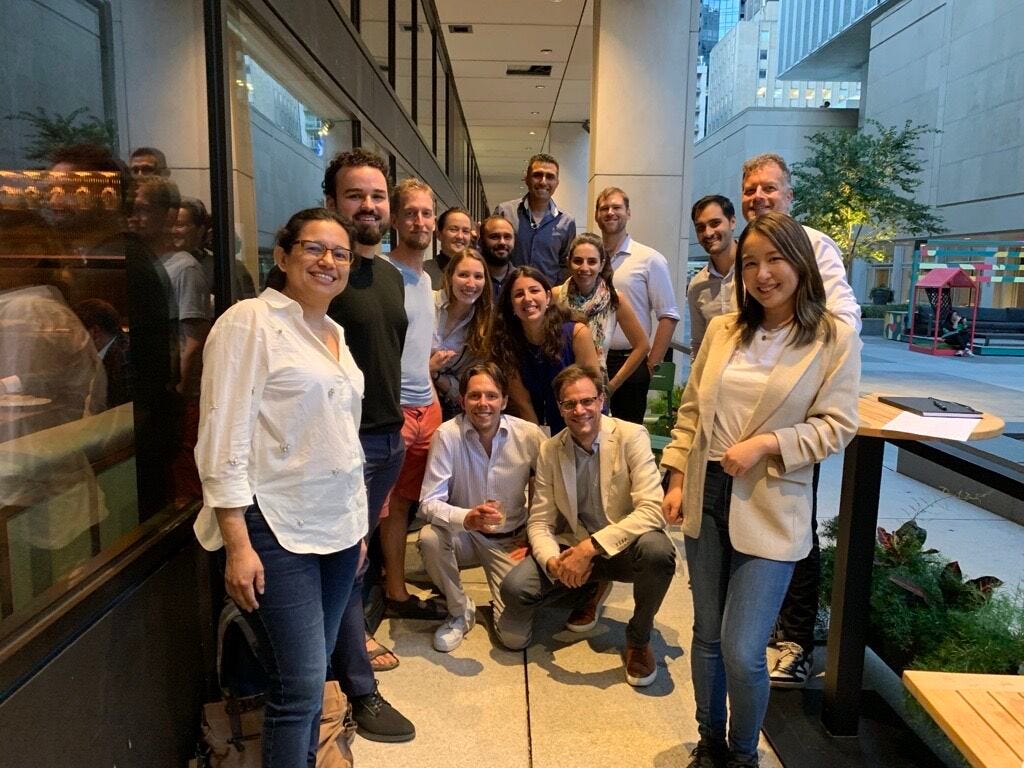